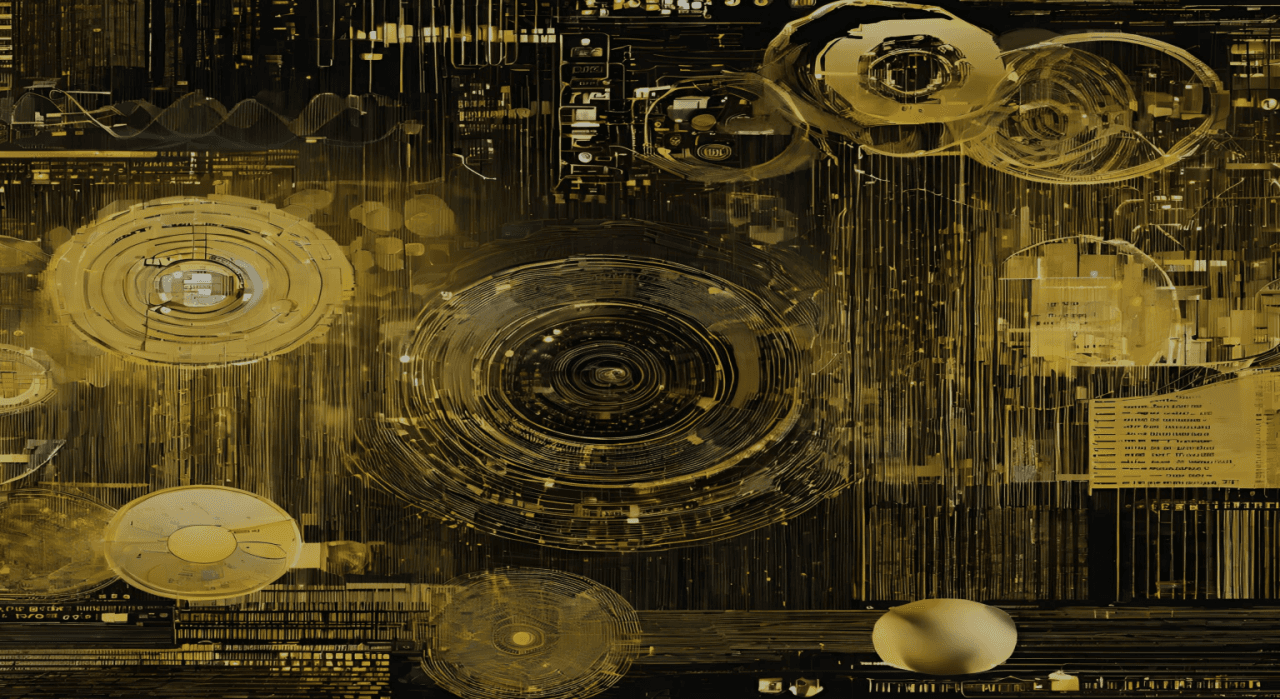
AI Generated
All that glitters is not gold vs All you need is data
Timeless adage warns us, All that glitters is not gold. This proverb, emphasizing the importance of looking beyond appearances, has resonated for centuries. However, in the era of AI, a different mantra seems to dominate:
- Bigger data, better results
- Random sub-sampling represents the statistics
- All you need is data
Big models are computationally expensive and are prone to overfitting. From our experience a bigger model makes sense when considering a very complex problem with a lot of features and data, a bigger model might be necessary to capture the underlying relationships. Big models are data hungry, they need a lot of training data to perform well.
Random sub-sampling works when the Law of Large Numbers is applicable. Do we always have that luxury in the medical domain? The medical imaging data are processed by medical experts, radiologists, and histologists. We do have a shortage of radiologists, pathologists, 300-400 doctors only in US commit suicide annually [1]. From our point of view it is better to work with small, well-designed core-sets. Another major drawback is representativeness. If the population has subgroups with distinct characteristics, a small random sample might not capture the variability within those subgroups. Therefore, focusing on well-designed core sets, even if smaller, becomes a more practical approach.
A fancy model is only as good as the data you train it on. Random splits can sometimes leave you with training on apples and testing on oranges! Instead from our experience it is better to systematically design the training and testing datasets, instead of randomly splitting.
All you need is data – data can be noisy, and do not contain additional information. AI extracts information from the data, hence bad data can lead to bad decisions. Collecting, storing, and labeling massive datasets is a huge financial burden especially in the medical domain.
The AI era cliches result in high development costs of the AI solutions.
All that glitters is not gold.
[1] Tamás Landesz, Sangeeth Varghese, Karine Sargsyan, Future Intelligence: The World in 2050-Enabling Governments, Innovators, and Businesses to Create a Better Future, Springer International Publishing. 10.1007/978-3-031-36382-5_5.