No cancer is the same: Enter AI, empowered by data analysis!
What is Cancer?
Normally, our bodies tightly regulate cell division. Cells grow and divide to replace old or damaged ones, but when they die, they are supposed to be cleaned up by the body.
Presence of cancer leads to the disruption of the above mentioned process. Cells keep dividing even when they shouldn't, forming a mass called a tumor. There are two main types of tumors:
According to [1] cancer will cost the world $25 trillion over the next 30 years and there are 5 cancer types that will account for half of the global cost.
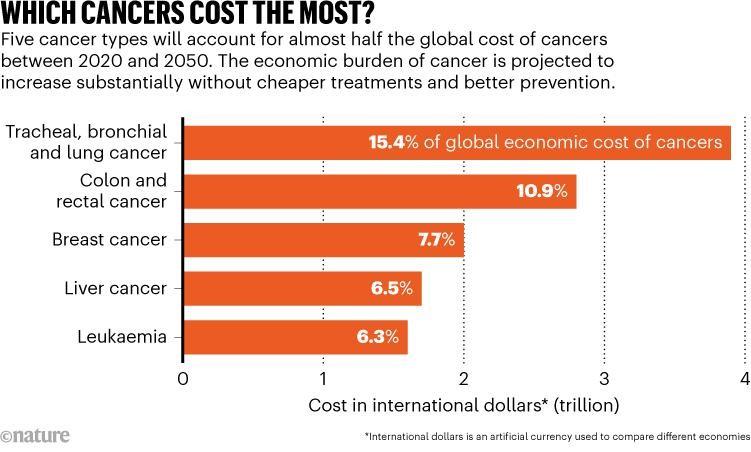
https://www.nature.com/articles/d41586-023-00634-9
- Benign tumors: These are not cancerous. They don't spread to other parts of the body and are usually not life-threatening. However, depending on their location and size, they can sometimes cause problems or require removal.
- Malignant tumors: These are cancerous. Malignant tumors can invade nearby tissues and have the potential to spread (metastasize) through the bloodstream or lymphatic system to other parts of the body. This spread is what makes cancer so serious.
Breast cancer is the second leading cause of cancer death among women, after lung cancer [cancer.org]. In 2020, there were an estimated 2.3 million new cases diagnosed worldwide. Early detection is crucial in fighting breast cancer, and regular mammograms are the best way to achieve this. According to Rodriguez et al. [2] the mean AUC of the radiologists was 0.814, while AI based commercial system AUC was reported 0.84. Therefore the research of cancer still has significant importance.
There are 5 open source mammography datasets CBIS-DDSM [3], InBreast [4], CSAW-M [5], KAU-BCMD [6], VinDr-Mammo [7].
In the current article we provide analysis of CBIS-DDSM based dataset. The dataset include region of pathology(ROI), descriptors for mass shape, mass margin, calcification type, calcification distribution, breast density, overall BI-RADS assessment from 0 to 5; rating of the subtlety of the abnormality from 1 to 5.
There are two main approaches found in literature to detect the pathology: analyze the mammogram image or analyze the pathology region only. Here we provide analysis based on the first approach.
Cancer is a highly heterogeneous disease. That means even within a specific type of cancer, tumors can have unique characteristics. Here we analyze the cases when the model fails to classify benign vs malignant.
The dataset contains mass and calcification abnormalities.
The initial dataset contains 3568 records annotated separately as calcification and masses, however there are duplicates.
Before cleansing
- mass abnormalities in training set are 1318
- mass abnormalities in testing set test are 378
- calcification in train set are 1546
- calcification in test are 326
After removing identical records:
- mass abnormalities in training set are 1231
- mass abnormalities in testing set test are 361
- calcification in train set are 1227
- calcification in test are 38
Below is 2D feature space analysis through PCA for training and testing sets.
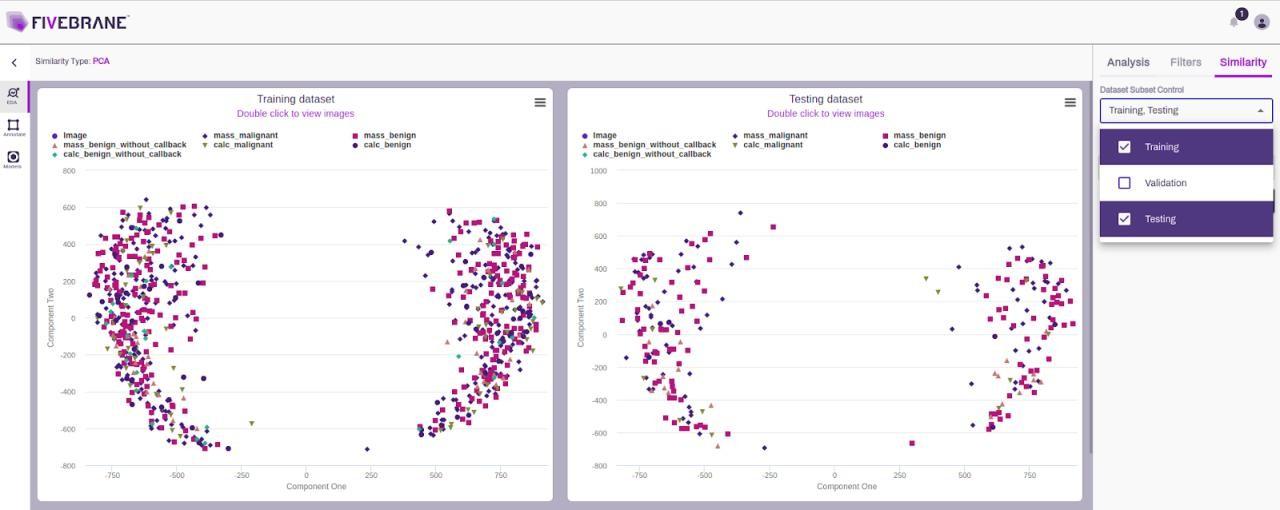
Breast Cancer PCA for CBIS-DDSM dataset
In the figure below the distributions of images are shown per different classes for both training and testing datasets.While the table summarizes the cases assessment distributions and also its representation in the training dataset.
There is a good overview about the different neural networks and approaches to train the dataset here [8].
The authors show the baseline accuracy with transfer learning is 61.80 with MobileNetV2, which they have improved 5.6% and achieved 67.4%.
We have trained the model with a cleaned dataset to reduce the early overfitting scenario likelihood and used ResNet50.
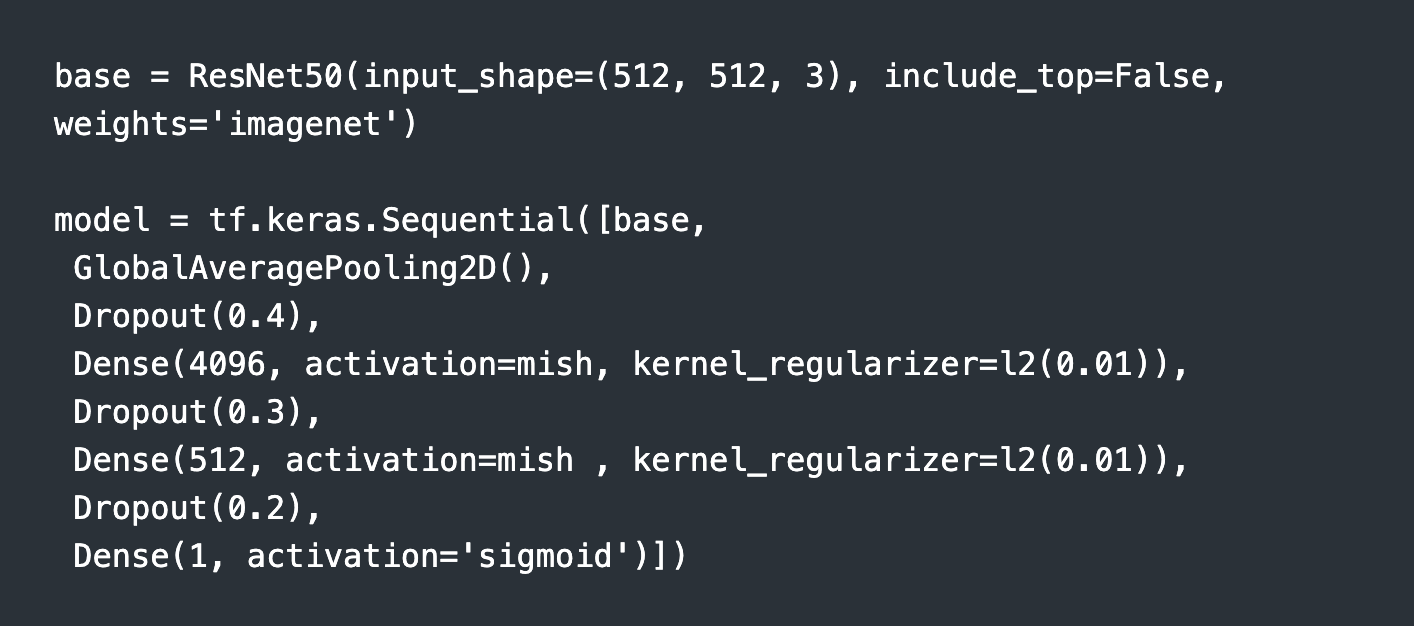
With this network we have combined both fine tuning and transfer learning approaches with 512x512 images size. The best registered performance is 75.8 F1 score, with 72.25% accuracy and 72% recall. Used RMSprop optimizer
- Fine tuned with 0.001 learning rate for 10 epochs
- Loaded the fine tuned network, unfrozen the whole network and trained with 0.0001 learning rate.
Overall we have 235 benign cases and 164 malignant cases in the testing set
In the figure below the distributions of images are shown per different classes for both training and testing datasets.While the table summarizes the cases assessment distributions and also its representation in the training dataset.
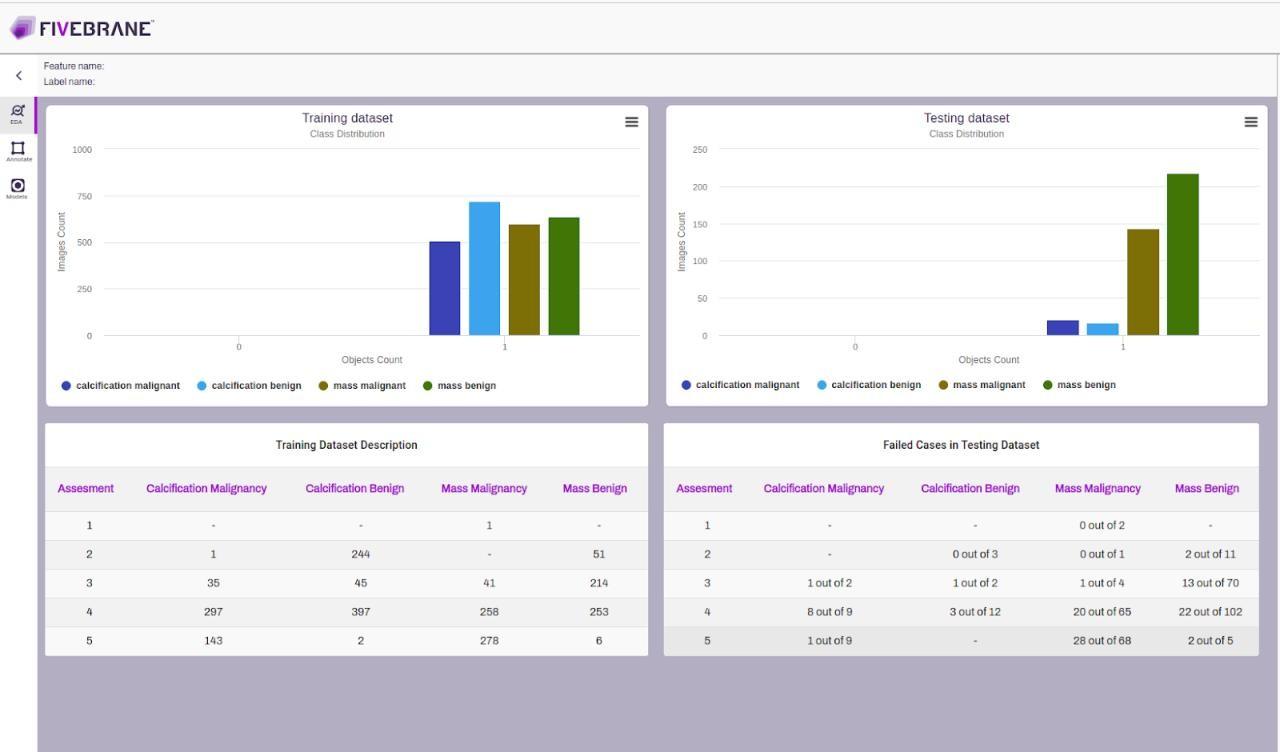
Here we see that the less represented case in training dataset, that is calcification malignant has higher failure rate 50%, we have 10 correct predictions from 20, the most represented case calcification benign is less represented in testing set, according to data we can say that its failure rate is 23%, and due to the data scarcity the uncertainty is high. The next well represented case in the training dataset is mass benign for which we see 20.7% failure rate.
From the above mentioned results it is clear that for robustness and reliability of the AI model performance it is crucial to thoroughly design training and testing datasets, keeping in mind that sufficiently large statistics is also crucial for reduction of the results uncertainty.
Punitha et al. in [9] have shown a multistage breast cancer detection method which achieved 98.1% accuracy and 97.8% for recall (sensitivity and specificity). The authors used texture features extracted through Gray Level Co-occurrence Matrix and Gray Level Run-Length Matrix. It has its own drawbacks because of its dependency on early stage phase accuracies. However, it means that radiomics features play a significant role in the analysis and during development we should take that into account properly. It is another topic that we will discuss later.
[1]. Freda Kreier, Cancer will cost the world $25 trillion over next 30 years
[2]. A. Rodriguez-Ruiz, K. Lång, A. Gubern-Merida, M. Broeders, G. Gennaro, P. Clauser, T. H. Helbich, M. Chevalier, T. Tan, and T. Mertelmeier, ‘‘Stand-alone artificial intelligence for breast cancer detection in mammography: Comparison with 101 radiologists,’’ J. Nat. Cancer Inst., vol. 111, no. 9, pp. 916–922, 2019
[3]. R. S. Lee, F. Gimenez, A. Hoogi, K. K. Miyake, M. Gorovoy, and D. L. Rubin, "A curated mammography data set for use in computer aided detection and diagnosis research", Sci. Data, vol. 4, no. 1, pp. 1–9, Dec. 2017
[4]. I. C. Moreira, I. Amaral, I. Domingues, A. Cardoso, M. J. Cardoso, and J. S. Cardoso, "INbreast: Toward a full-field digital mammographic database", Acad. Radiol., vol. 19, no. 2, pp. 236–248, 2012
[5]. M. Sorkhei, Y. Liu, H. Azizpour, E. Azavedo, K. Dembrower, D. Ntoula, A. Zouzos, F. Strand, and K. Smith, "CSAW-M: An ordinal classification dataset for benchmarking mammographic masking of cancer", in Proc. Neural Inf. Process. Syst. Track Datasets Benchmarks, vol. 1, 2021, pp. 1–12
[6]. A. S. Alsolami, W. Shalash, W. Alsaggaf, S. Ashoor, H. Refaat, and M. Elmogy, "King abdulaziz university breast cancer mammogram dataset (KAU-BCMD)", Data, vol. 6, no. 11, p. 111, Oct. 2021
[7]. H. H. Pham, H. N. Trung, and H. Q. Nguyen, "VinDr-Mammo: A largescale benchmark dataset for computer-aided detection and diagnosis in full-field digital mammography (version 1.0.0)", PhysioNet, pp. 1–11, Jan. 2022
[8]. A. Jaamour, C. Myles, A. Patel, Shuen-Jen Chen, L. McMillan ,D. Harris-Birtill, "A divide and conquer approach to maximize deep learning mammography classification accuracies", https://doi.org/10.1371/journal.pone.0280841, May-2023
[9]. Punitha S., Amuthan A. and Joseph K.S., 2018. Benign and malignant breast cancer segmentation using optimized region growing technique. Future Computing and Informatics Journal, 3(2), pp.348–358. Dec. 2018